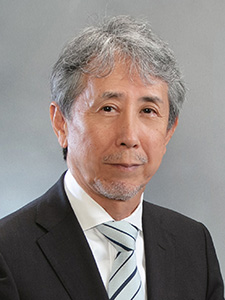
“Hierarchical modular reinforcement learning for metacognition”
Dr. Mitsuo Kawato
Director, ATR Brain Information Communication Research Laboratory Group
Date/Time
Monday, October 31 2022, 13:30-14:20
Abstract
Computational learning theory asserts that a machine learning algorithm necessitates more training samples than the number of its learning parameters for generalization. Accordingly, deep neural networks use tens of millions training samples. Yet, our brain contains tens of billions neurons and even more synapses, but we typically learn new behaviors within tens to hundreds of trials. Drastic dimension reduction should take place in the brain to explain these tremendous contrasts. We proposed that synchronization of firing, compartments, internal models, parallel and hierarchical modules, and higher cognitive functions may all contribute to this dimension reduction. By extending fMRI decoded neurofeedback paradigms (Shibata et al., 2011, 2018), Kawato and Cortese (2021) demonstrate that hierarchical and modular reinforcement learning architecture is a key for learning from a small sample, and it provides a neural mechanism of metacognition. Cognitive reality monitoring network (CRMN) comprises of parallel and layered, generative-inverse model pairs and their gating network in the prefrontal cortex. This model was motivated by “perceptual reality monitoring” of Hakwan Lau and its GAN extension by Samuel Gershman. Based on mismatches between computations by generative and inverse models, as well as reward prediction errors, CRMN computes a “responsibility signal” that gates selection and learning of pairs in perception, action, and reinforcement learning. A high responsibility signal is given to the pairs that best capture the external world, that are competent in movements (small mismatch), and that are capable of reinforcement learning (small reward-prediction error).